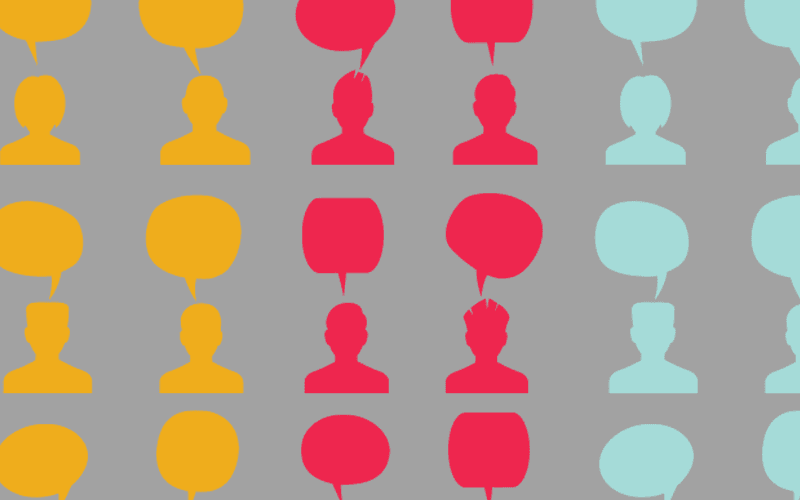
There’s no questioning the impact of deeper member engagement. Members are more easily retained. They give larger gifts, or just give in general. They are more likely to serve as volunteers. Engaged members are more active evangelists of your organization.
There’s also no questioning the fact that we all have limited resources, among many other challenges, that make the task of actively engaging members one on one seem daunting, if not impossible. Segmentation is a proven method, but if you’ve never tried it before, if can be quite intimidating.
While it’s a challenge to get started, the key is just to start. The good news is that you don’t have to be perfect initially. Anything is better than nothing when it comes to analyzing your membership data.
Here’s a suggestion for how to get started.
Since we can’t effectively engage every member one on one, try to categorize members into buckets based on their current temperature. A great tool for this is Net Promoter Score (NPS). NPS is a simple survey where you ask your members one question: On a scale of 0 to 10, how likely would you be to recommend our products and services to your friends? Based on responses, you can quickly categorize members into three buckets: Promoters, Passives, and Detractors.
Make the information more actionable by asking an additional, mission-oriented question, such as “Rate your connection to our organization’s mission.” With responses to these two questions, you can refine your categories and ID your most connected members.
Next, plan your strategy for engaging members in the various groups. Develop a compelling story that connects your Prime Targets with your mission. Show your “at risk” members how you are listening and making improvements based on their feedback. And, at all costs, get those members in your “the future” bucket more involved!
Subscribe to our blog
Curated trends and resources, right to your inbox.